A hint to The Book of Why by Judea Pearl
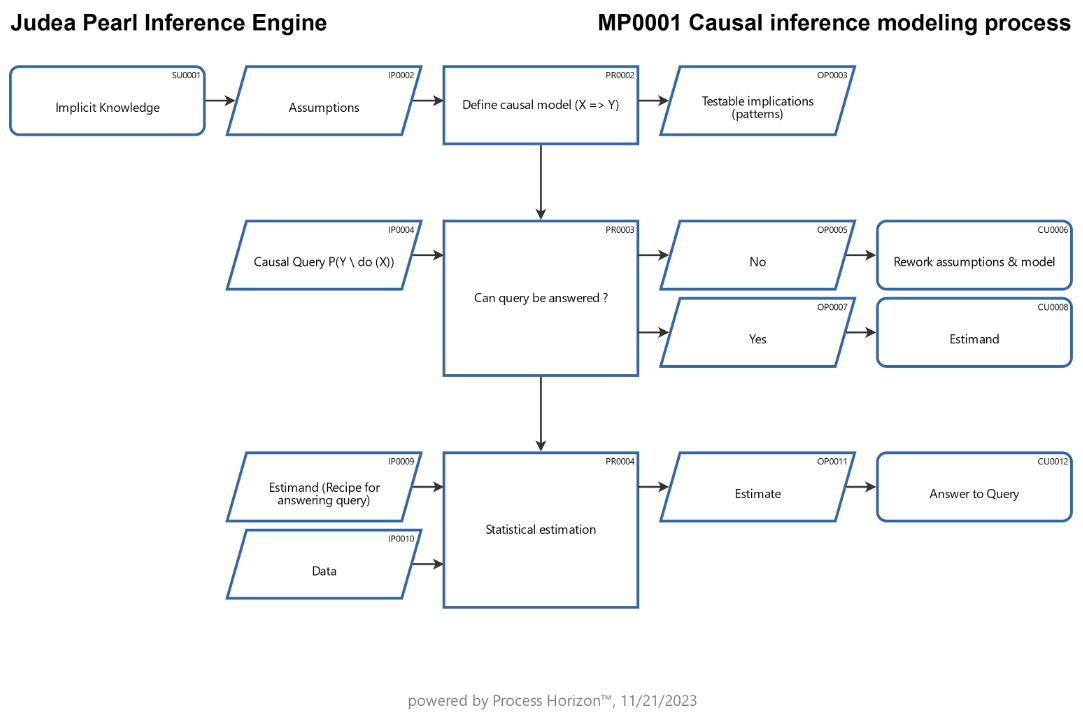
The Book of Why by Judea Pearl is a seminal work on causal inference, and it introduces the concept of a causal inference engine based on the framework of causal diagrams (also known as causal graphs (X => Y).
Here's a simplified explanation of the causal inference process according to Pearl's approach:
1. Modeling Causal Relationships using Causal Diagrams
- Nodes and Arrows: Identify the variables of interest and represent them as nodes in a causal diagram. Arrows between nodes indicate causal relationships.
- Directed Acyclic Graphs (DAGs): Ensure that the causal diagram forms a Directed Acyclic Graph, meaning there are no cycles in the arrow connections. This helps avoid logical contradictions in the causal relationships.
2. Convert Assumptions into Causal Statements
- Structural Causal Model (SCM): Translate the causal diagram into a Structural Causal Model, where each variable has a structural equation describing how it depends on its direct causes.
- Counterfactuals: Define counterfactuals, which involve asking "What if?" questions about the potential outcomes if certain interventions or changes occurred.
3. Causal Inference Using Do-Calculus (P(Y \ do (X))
- Interventions and Counterfactuals: Pearl introduces the concept of "do-calculus," a set of rules for manipulating the SCM to answer causal queries involving interventions (changes in variables) and counterfactuals (what would have happened under different conditions).
- Back-Door and Front-Door Criteria: Develop and apply criteria to identify "back-door paths" and "front-door paths" in the causal graph. These paths help determine which variables need to be controlled for to estimate causal effects accurately.
4. Quantifying Causal Effects
- Conditional Probability and Expectation: Express causal effects in terms of conditional probabilities and expectations. This allows for a quantitative understanding of the impact of interventions on the outcome variable.
- Potential Outcomes Framework: Leverage the potential outcomes framework to express causal effects in terms of the difference between what would happen under different treatment conditions.
5. Causal Inference in Practice
- Data and Parameter Estimation: Use observational or experimental data to estimate the parameters of the structural equations in the SCM.
- Sensitivity Analysis: Perform sensitivity analysis to assess the robustness of the causal inferences to different assumptions and potential sources of bias.
Pearl's approach to causal inference emphasizes the importance of modeling causal relationships explicitly, using causal diagrams and SCM. The do-calculus provides a systematic way to reason about causation and make inferences from observational or experimental data. This framework has had a significant impact on the field of causal inference and has influenced the development of methodologies for extracting causal knowledge from data.
What are its implications on the EUAIact & AIBillofRights ?