Why is AI explainability crucial to fostering trust in AI ?
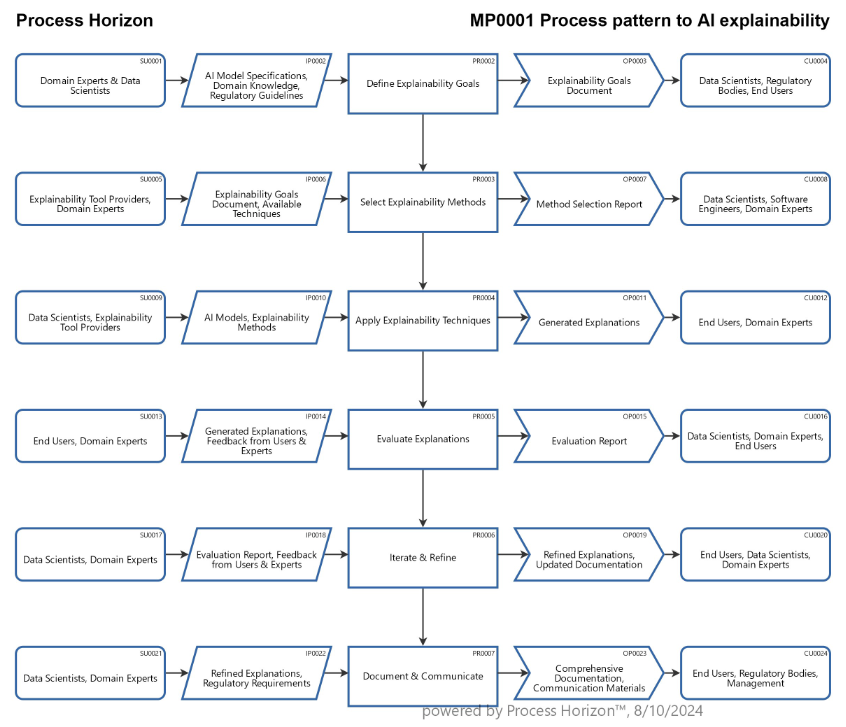
1. Transparency
Explainable AI provides transparency into how decisions are made by algorithms. When users understand the rationale behind an AI system’s output, it demystifies the process and helps build confidence in its results.
Transparency allows stakeholders to see the inner workings of AI models, making it easier to identify and address any issues or biases. This openness is key to trust as it assures users that the AI behaves in a predictable and understandable manner.
2. Accountability
When AI decisions can be explained, it is easier to hold the system accountable for its actions. If an AI system makes a mistake or produces an undesirable outcome, having a clear understanding of how the decision was reached helps in identifying and rectifying the issue.
Accountability ensures that AI systems are not black boxes. This is essential for users and organizations to trust that the AI is functioning correctly and ethically. It also helps in assigning responsibility when errors occur.
3. Bias & Fairness
Explainability helps in detecting and mitigating biases in AI algorithms. By understanding how a model arrives at its decisions, it is possible to identify if the model is unfairly biased against certain groups or individuals.
Ensuring fairness and minimizing bias are crucial for ethical AI use. Users are more likely to trust AI systems that are fair and unbiased. Explainability helps in scrutinizing and improving the model to ensure equitable outcomes.
4. Regulatory Compliance
Many regions have regulations that require AI systems to be explainable, especially in sectors like finance and healthcare. These regulations are designed to protect users and ensure that AI systems operate within legal and ethical boundaries.
Compliance with regulations not only helps avoid legal repercussions but also builds trust with users who are concerned about their rights and the ethical use of AI. It demonstrates that the organization is committed to responsible AI practices.
5. User Empowerment
When AI systems provide explanations, users can make more informed decisions based on the AI’s outputs. Understanding the reasoning behind AI recommendations or decisions enables users to assess the relevance and accuracy of the information.
Empowered users are more likely to trust and effectively use AI systems. Explainability helps users feel confident in integrating AI insights into their own decision-making processes.
6. Debugging & Improvement
Explainable AI helps developers and data scientists understand how models are performing and why they may be producing certain results. This understanding is crucial for debugging and improving the system.
Continuous improvement and accurate performance tuning are essential for maintaining trust in AI systems. Explainability allows for iterative enhancements based on insights derived from model behavior.
7. Ethical Considerations
Explainability aligns with ethical principles by ensuring that AI systems operate transparently and are understandable to those affected by their decisions. It reflects a commitment to ethical AI practices.
Ethical AI fosters trust among users who are concerned about the moral implications of AI decisions. Explainable systems support ethical decision-making and promote responsible AI development.
8. Enhanced Decision-Making
Explainable AI helps users understand how specific inputs lead to outputs, which can improve their overall decision-making process by providing clarity on the factors influencing the results.
Enhanced decision-making capabilities lead to better user experiences and outcomes. When users trust the AI's explanations, they are more likely to use its outputs effectively in their own decision processes.
These factors can collectively ensure that AI systems are reliable, understandable and trustworthy. Explainability of AI algorithms is thus fundamental for building and maintaining trust.
>>> AI trust by stakeholder oriented process design of AI algorithms
Using the following link you can access this sandbox SIPOC data & process model in the ProcessHorizon web app and adapt it to your needs (easy customizing) and export or print the automagically created visual AllinOne process map as a PDF document or share it with your peers: https://app.processhorizon.com/enterprises/jC7URdpzPaLgqSxLR81DHMVs/frontend