Process Horizon AI process flywheel
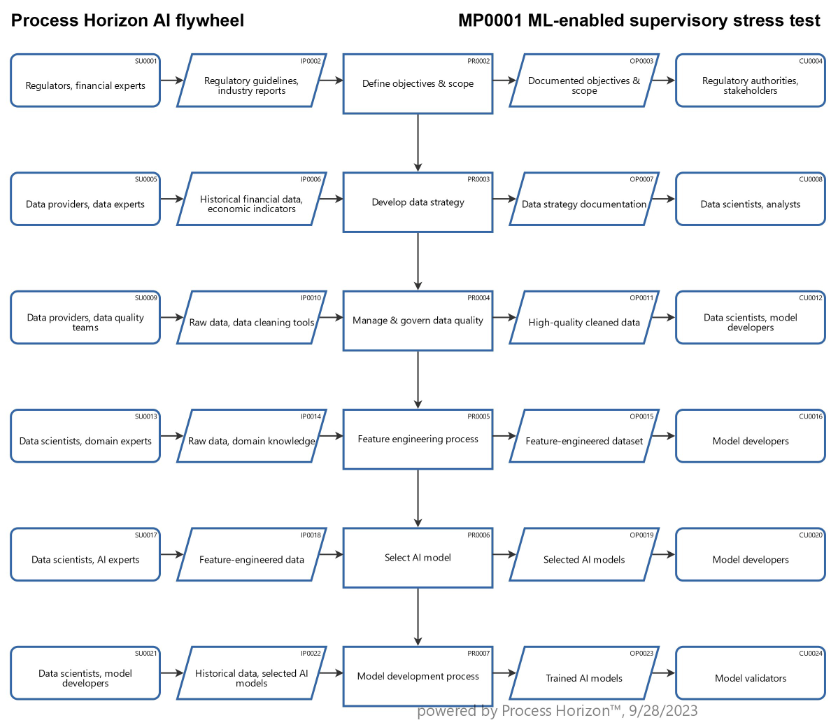
Successful deployment of an AI machine learning enabled supervisory stress test for systemically important banks depends on an ideation process as follows to guide the development of such an AI-driven stress test:
1. Establish clear objectives & scope
- Define the primary objectives of the AI-enabled stress test, such as assessing capital adequacy, systemic risk, or identifying vulnerabilities. Clearly delineate the scope of the test.
2. Develop data strategy
- Develop a comprehensive data strategy to collect, preprocess, and manage the necessary data. This may include financial data, market data, economic indicators, and bank-specific information.
3. Manage & govern data quality
- Ensure data quality and governance by implementing data cleaning, validation, and maintenance procedures. Data should be accurate, reliable, and up-to-date.
4. Feature engineering process
- Identify relevant features or variables that will be used as inputs to the AI models. This may involve domain expertise and data analysis to select the most informative features.
5. Select AI model
- Choose appropriate AI and machine learning algorithms for stress testing. Common choices include neural networks, decision trees, ensemble methods, and time series forecasting models.
6. Model development process
- Develop and train AI models using historical data. Models should simulate the bank's balance sheet, income statement, and risk exposures under different stress scenarios.
7. Scenario generation process
- Utilize AI techniques to generate a wide range of realistic stress scenarios, including both historical and hypothetical events. These scenarios should reflect extreme but plausible economic conditions.
8. Scenario calibration process
- Calibrate the severity and duration of stress scenarios based on historical data, expert opinions, and AI-driven analysis of extreme events.
9. Capital adequacy assessment process
- Use AI models to calculate capital requirements and assess the impact of stress scenarios on regulatory metrics like CET1 capital ratios, leverage ratios, and risk-weighted assets.
10. Risk assessment process
- Employ AI to analyze the impact of stress scenarios on various risk factors, such as credit risk, market risk, liquidity risk, and operational risk. Machine learning can help identify hidden patterns and correlations.
11. Model validation process
- Independently validate AI models and stress scenarios to ensure accuracy and reliability. This may involve back-testing against historical crises.
12. Ensure explainability & transparency
- Ensure that AI models are interpretable and transparent. Develop methods to explain model predictions and outcomes to regulators, banks, and stakeholders.
13. Regulatory action planning
- Determine regulatory actions based on AI-driven stress test results, including capital adequacy requirements, risk management improvements, or supervisory interventions.
14. Supervisory collaboration process
- Collaborate with other regulatory bodies and international counterparts to ensure consistency in AI-driven stress testing methodologies and results.
15. Crisis management planning
- Develop plans for managing a crisis if AI-driven stress test results indicate that a bank is at risk. Ensure coordination with other regulators and resolution strategies.
16. Ensure scalability & flexibility
- Ensure that the AI-driven stress testing framework is scalable and flexible enough to adapt to evolving risks and market dynamics.
17. Establish regular testing cycle
- Establish a regular schedule for conducting AI-driven stress tests, taking into account the fast pace of change in financial markets.
18. Review & adapt framework
- Periodically review and adapt the AI-enabled stress testing framework to incorporate new AI technologies, emerging risks, and lessons learned from previous tests.
19. Maintain documentation & reporting
- Maintain detailed documentation of the AI-driven stress testing process and results. Provide comprehensive reports to relevant stakeholders.
20. Ethical & legal considerations
- Address ethical and legal considerations related to the use of AI, including data privacy, bias, and transparency.
Using the following link you can access this sandbox process model in the ProcessHorizon web app and adapt it to your needs (easy customizing) and export or print the automagically created visual process map as a PDF document or share it with your peers: https://app.processhorizon.com/enterprises/s4uHs1Ho7K3pzySkuywx13N9/frontend